AI for CAE
The question isn’t “if” but “how soon”
CAE, or Computer-Aided Engineering (CFD, FEA, FSI, and optimization), is a field that utilizes computer software and tools to deliver the design, analysis, and optimization of engineering systems and components. This field plays a crucial role in industries such as automotive, aerospace, manufacturing, and civil engineering, enabling engineers to make informed decisions and improve the efficiency and reliability of their designs.
With recent developments, seeing how AI (Artificial Intelligence – here I mean rather Machine Learning but only calling it AI to make things easier) is disrupting field by field, everything seems that AI will disrupt the CAE field too. In my humble opinion, it’s just a matter of time and the only question is when (and how exactly) it happens rather than if it happens.
We’re just somewhere at the beginning and it’s even very difficult to say what is going on at the moment – not a mention that it’s impossible to predict what will happen in the future. However, there are a couple of things that we CAN say.
The thing is, the usual CAE workflow involves a bunch of easy manual tasks and decisions based on data. And AI seems to be perfect for lending a hand with all of that.
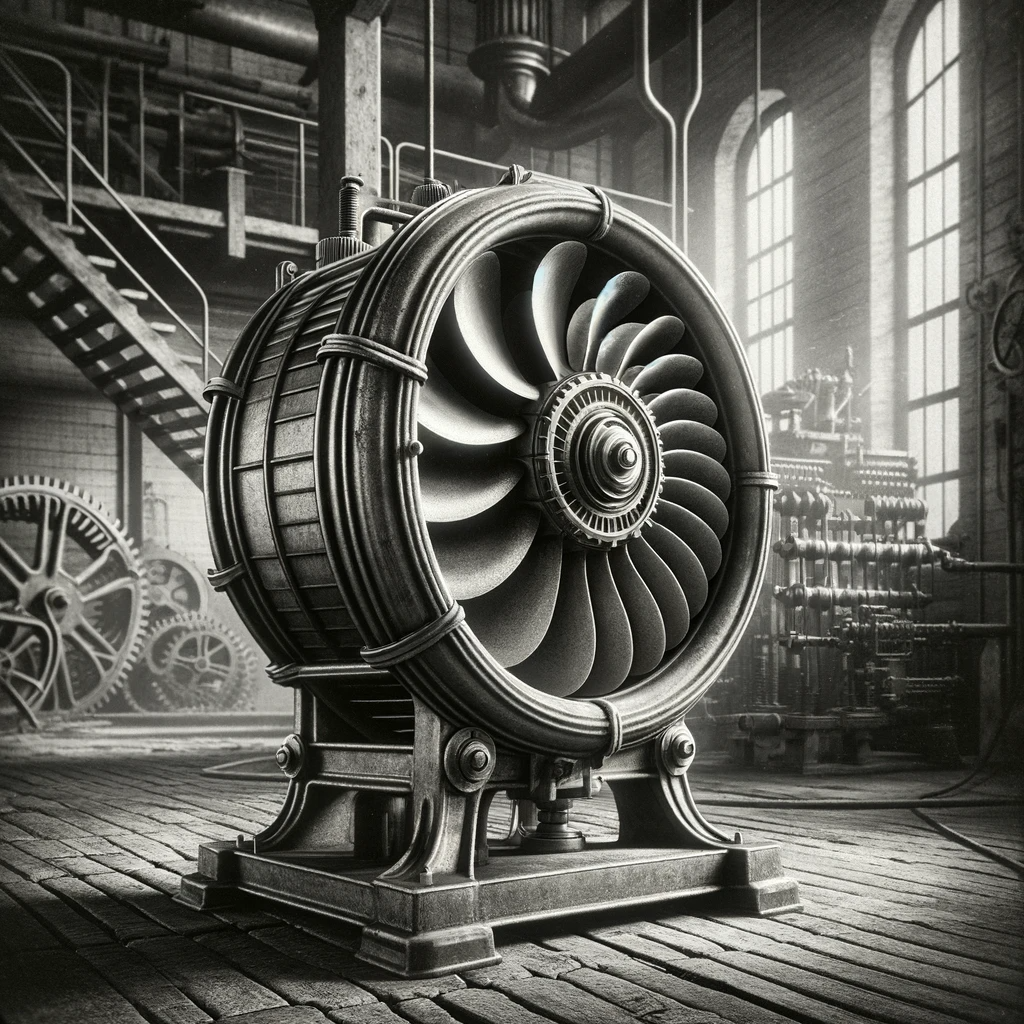
History of computational-aided industrial designs
CAE is a relatively new discipline with drastic development. Let’s go through an example of how time went by using the example of industrial fan designs. An industrial fan is a very widespread machine that has the task of transporting air through pipes from place to place. It’s being used in every industrial field.
The history of industrial fan design has evolved significantly over time, reflecting advancements in CPU power and technology, especially, an understanding of aerodynamics and the rise of Computational Fluid Dynamics (CFD). We had a couple of informal discussions with our industrial fan clients and here’s an overview of the key developments in terms of computation-aided design, sampled by decades:
Back in 1980, when a new fan was to be designed, the engineer had to calculate dozens of formulas manually (with a calculator), which resulted in the basic parameters of the fan. The newly designed fan was then manufactured, and tested and if it passed the test in order, it was possible to put it into production. For a single design engineer, the standard design time for a single new fan was several months or more (the designed fans had to be physically tested).
In 1990, simple computer programs were commonly used instead of a calculator, i.e. a series of simple subsequent calculations, 1D simulations. When Microsoft Excel came along it helped with clarity and simplicity. Guess, feel and experience still prevailed over brute computing power. A single engineer could make one complex design per month.
By 2000, there were already 2D CFD simulations that brought more profound knowledge about the flow field, improving aerodynamic efficiency. A few comprehensive proposals per month and fewer physical tests were needed.
In 2010, 3D CFD simulations, comprehensive knowledge of the flow field, sophisticated aerodynamics, multiple simulations and optimization, and the possibility of choosing from several candidates for production and eventual testing. 3D CFD did not speed up the design process but brought very accurate results and a complex understanding of the flow field and so much less physical tests were needed.
By 2020, the design process had become fully automated and embraced full-scale optimization. A single engineer could run hundreds or even thousands of simulations per month and the fan design process goes completely virtual without the need for physical measurements.
Now it’s January of 2024 and it seems that the pace of change only accelerates.
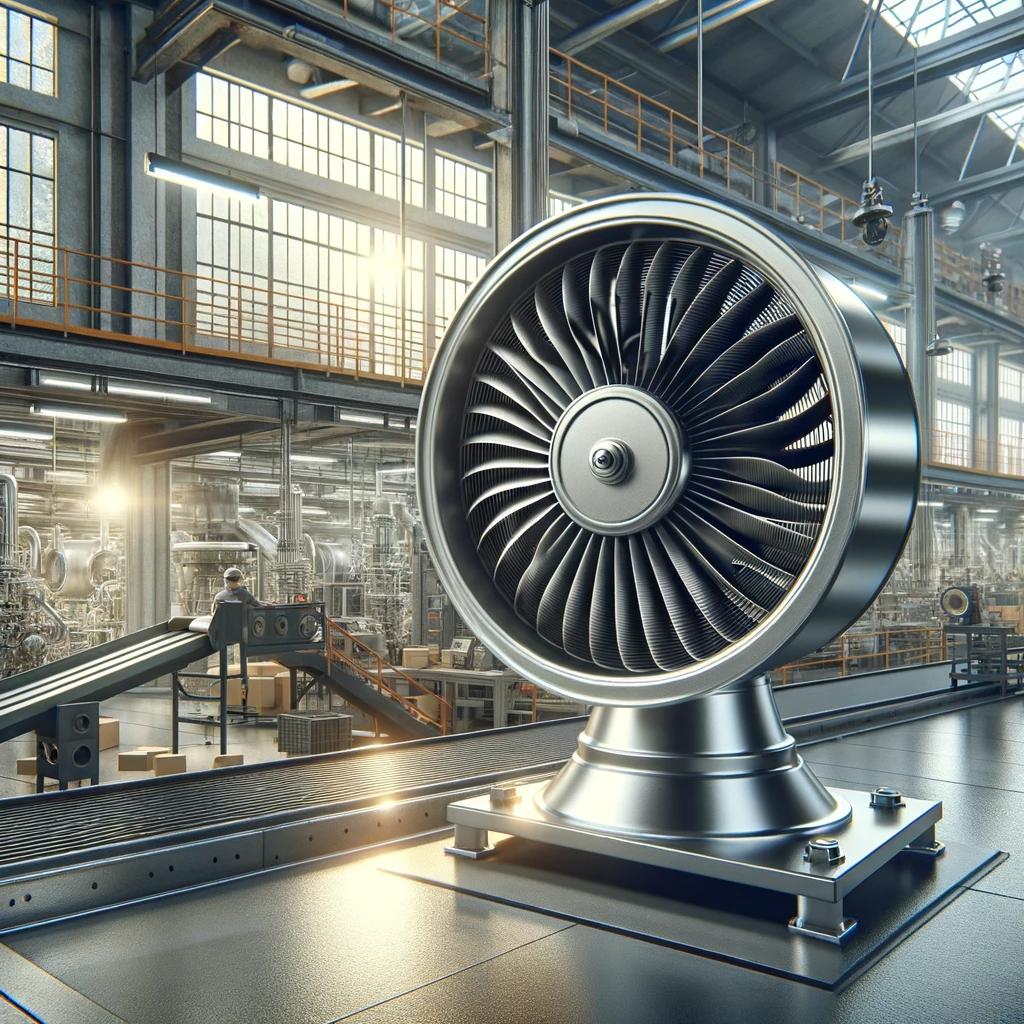
Rise of AI in the early 20s
Large Language models broke the ice and AI becomes more integrated into various aspects of everyday life. The current era of AI is dominated by deep learning, a type of machine learning involving deep neural networks. Breakthroughs in this field have led to remarkable advancements in image and speech recognition, natural language processing, and autonomous vehicles. The availability of big data and continued exponential growth in computing power have driven these advancements.
AI’s edge over humans
It’s been observed that the optimization is not intuitive at all. AI is infinitely superior to the human mind in terms of data analysis. AI can process vast amounts of data and perform complex calculations much faster than humans. AI has virtually unlimited memory capacity, allowing it to store and retrieve vast amounts of information quickly and accurately. Humans, on the other hand, are limited by their cognitive abilities and can struggle to recall information with the same speed and accuracy.
AI systems can make decisions without being influenced by personal biases or emotions. This can lead to more objective and fair decision-making, where human biases can have negative consequences. AI systems can handle multiple tasks simultaneously without experiencing fatigue or loss of focus. Humans, on the other hand, have limitations in multitasking and can become overwhelmed or make mistakes when juggling too many tasks at once.
AI systems can be easily scaled up or down to meet the demands of a particular task or situation. They can handle large volumes of data and perform complex computations without significant performance degradation. Humans, on the other hand, may struggle to handle large-scale tasks or may require additional resources and time.
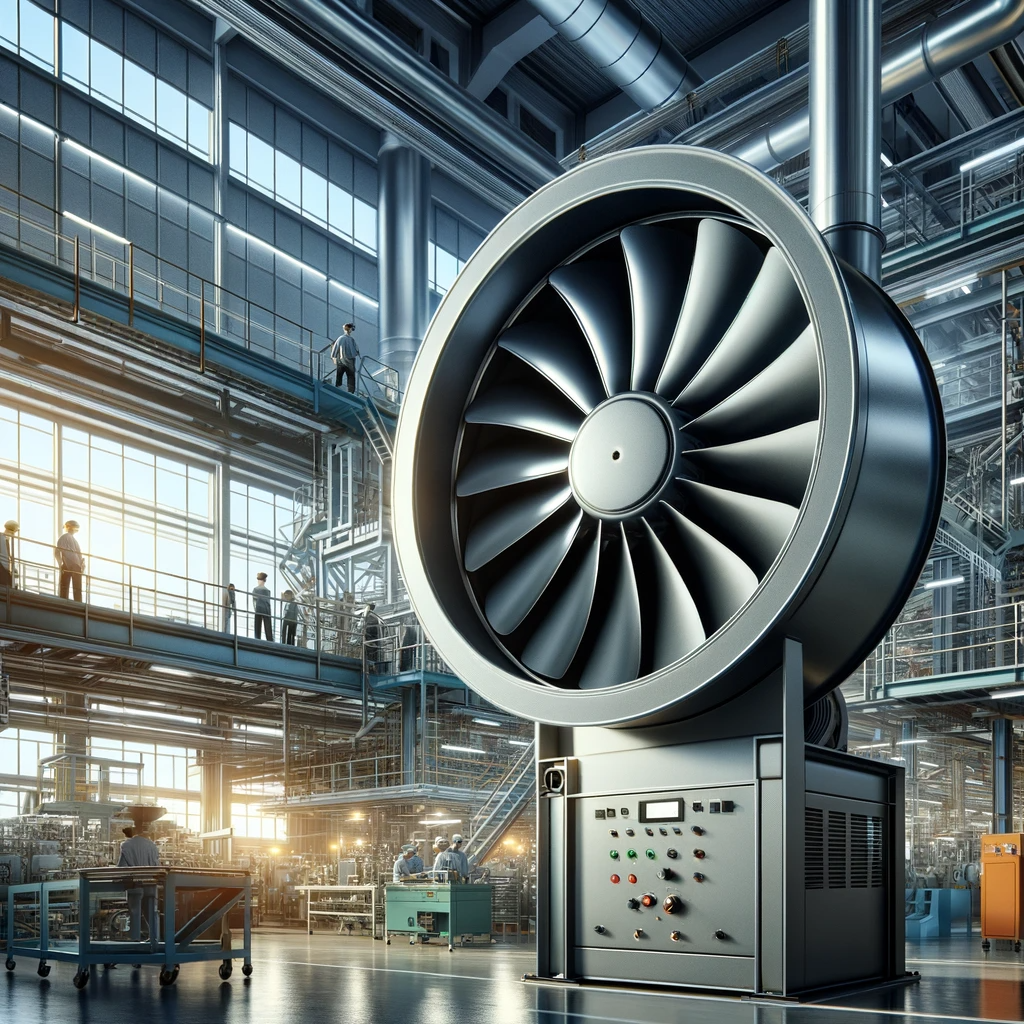
Human’s edge over AI
While AI has many advantages over humans in certain areas, it is important to note that humans still possess unique qualities. We humans can think creatively and come up with innovative ideas, think outside the box, and make connections that AI may not be able to make, especially in complex and interdisciplinary tasks.
Humans have a natural sense of common sense and intuition that allows them to make decisions based on their instincts and past experiences. Humans can adapt and learn from new situations and experiences. They can quickly adjust their thinking and behavior based on new information. AI, on the other hand, requires reprogramming or retraining to adapt to new situations.
We can solve complex problems by breaking them down into smaller parts and using critical thinking skills. AI may struggle with complex problem-solving that requires human-like reasoning and analysis. Humans can understand and interpret information within a broader context. They can understand the nuances and subtleties of language, culture, and social situations.
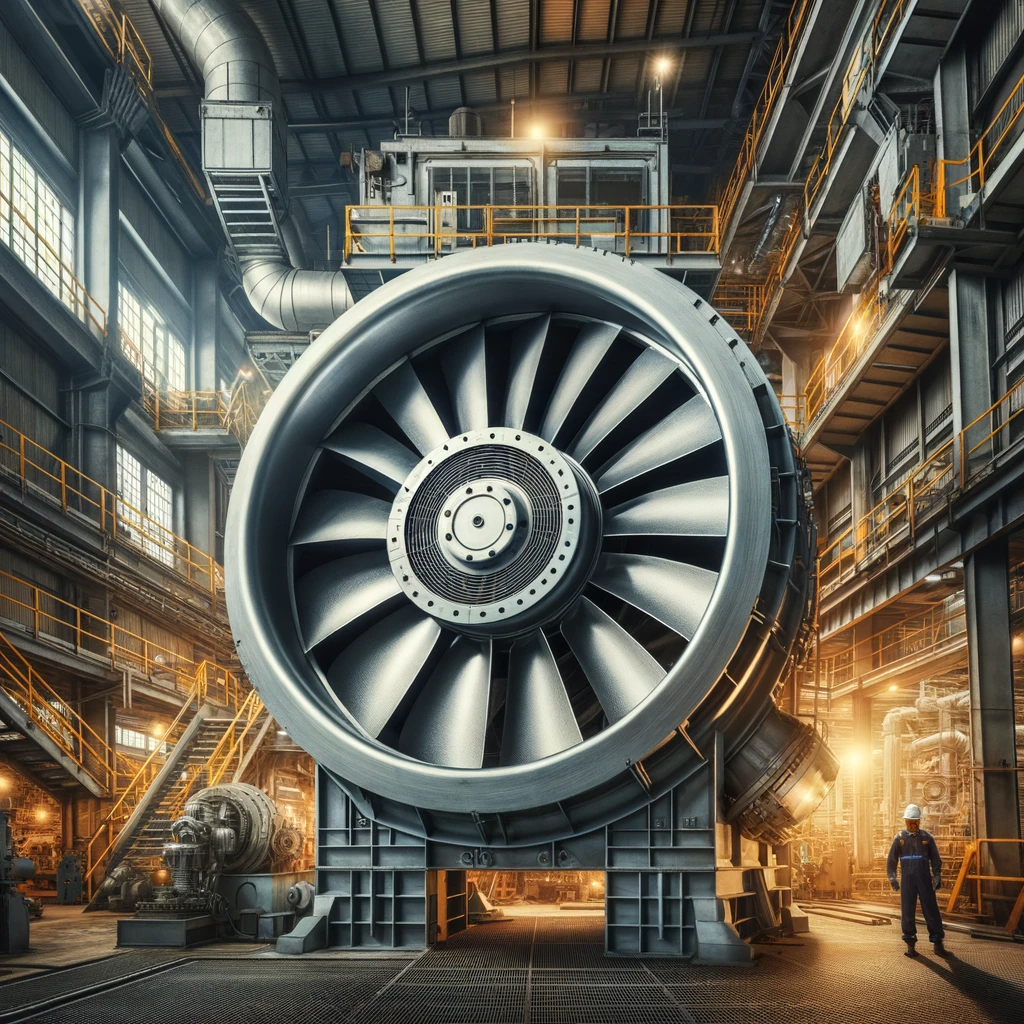
AI for CAE usage scenarios
The field of CAE is merely a field of playing with engineering data and AI has proved it’s great at playing with data. It seems that AI has great potential to revolutionize CAE by improving process speed, efficiency, and automation. Especially the process speed, because the simulation convergence typically takes a lot of time, and time is everything. The question is how to do that.
CAE processes are an extremely wide topic with many subsequent routines, let’s focus now only on AI for CFD simulations (FEA simulations follow similar principles) because the simulation time is the most expensive asset. The main issue at the moment is; at what level are we going to employ AI?
The first approach to the application of AI in CAE is in the optimization of design parameters, in other words, a special AI model (complex surrogate model) would completely replace the simulation runs. Let’s call it a surrogate approach. Standard simulations can be run multiple times with different design parameters to create the training data and write them into a special database. Large datasets of existing data (simulation results, measurements, baseline products, …) for a particular case, will be maintained in a special database. AI model could predict the new simulation-quality results by extrapolation of the database results. AI could do it in seconds while the proper simulation could do it in hours or days. This can help engineers and designers to quickly and efficiently find new designs based on their requirements. In this scenario, AI will be dealing with specific model parameters for a specific case (e.g. industrial fan) in a closed parametric space.
Benefits: Relatively straightforward to implement.
Drawbacks: Each database will be exclusive to a particular case it has been created for (the AI model created for the centrifugal fan wouldn’t work for Formula 1 aerodynamics). No exceptions. No lesson to take away.
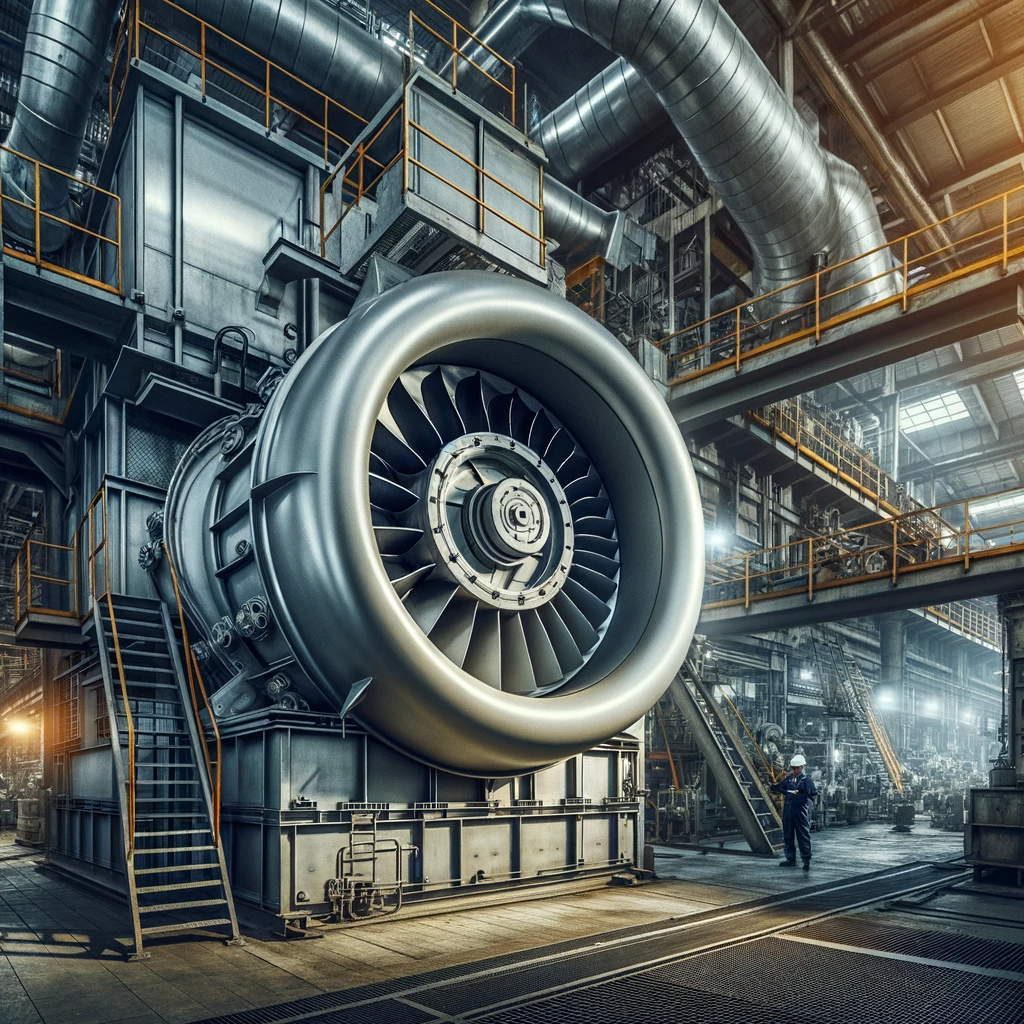
Another possible approach is the prediction of AI-modified fluid flow behavior. Let’s call it a flow field approach. AI algorithms can be trained on large datasets of various CFD simulations to learn patterns and correlations between different variables. This can enable predictions of fluid flow behavior in new scenarios, without the need for classical time-consuming simulations. There are many levels of freedom in this approach (a question of how general this approach should be). However, it is important to acknowledge that developing a robust and general enough AI model for this flow field approach presents significant challenges. Ensuring the model’s reliability and accuracy across a wide range of applications requires meticulous training and validation processes. The complexities of fluid dynamics together with the inherent variability in real-world industrial applications make it very difficult. At the moment it’s not very clear how to do this effectively. There is a wide range of possibilities from complete AI black-boxes to hybrid AI-aided CFD solvers.
Benefits: Usability on a wide range of applications.
Drawbacks: Extremely difficult. Not clear.
These two approaches differ in principle similar to the distinction between LLM (Large Language Model) and general AI (Artificial Intelligence). LLM refers to a language model that is designed to perform a specific task or application, while general AI refers to an AI system that is capable of performing a wide range of tasks and applications.
In summary, the choice between these two approaches boils down to the trade-off between specialization and versatility. While a single application may excel in its designated area, a general application offers the potential for broader applicability and scalability across a wide range of tasks.
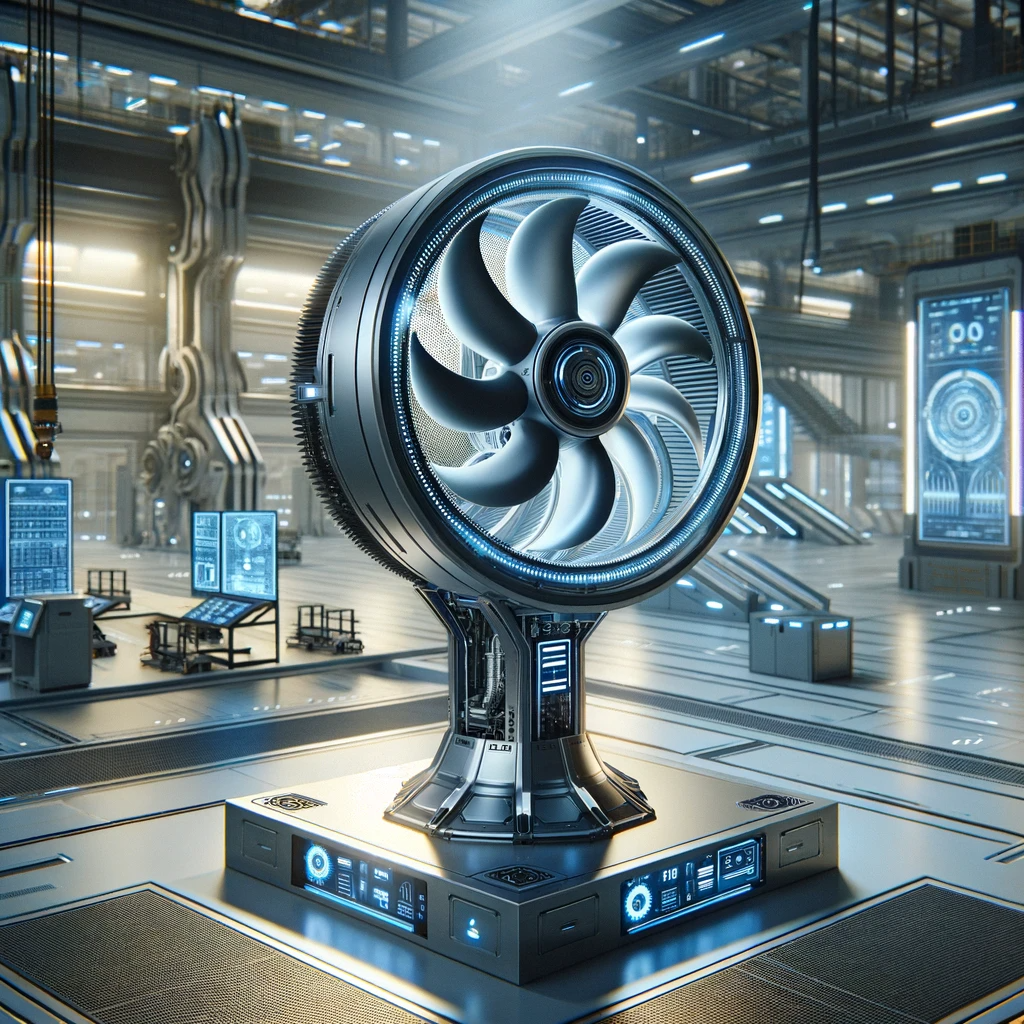
Training data is everything
Training data is absolutely critical when it comes to engineering simulations. It’s like the saying goes, “garbage in, garbage out.” If we don’t have high-quality and comprehensive training data, our AI-based models will be ineffective and unreliable. Unfortunately, over the past few decades, a significant amount of simulation data has been lost or discarded. This means that we’ve missed an opportunity on valuable lessons and insights that could have been taken from analyzing that data. That’s why one of our main challenges is to figure out how to efficiently save and store simulation data from now on. We need to make sure that we’re capturing and preserving this valuable information for future analysis and learning.
But it’s not enough to just store the data – we also need to know how to effectively mine and extract insights from it. By developing tools and algorithms that can operate on the data, we can uncover patterns, trends, and relationships that can inform the development of more accurate and efficient simulation models. The simulation data will be the foundation upon which future advancements in engineering simulation are built.
So, yes, training data is everything. It’s the key to unlocking the full potential of engineering simulations and driving progress in the field.
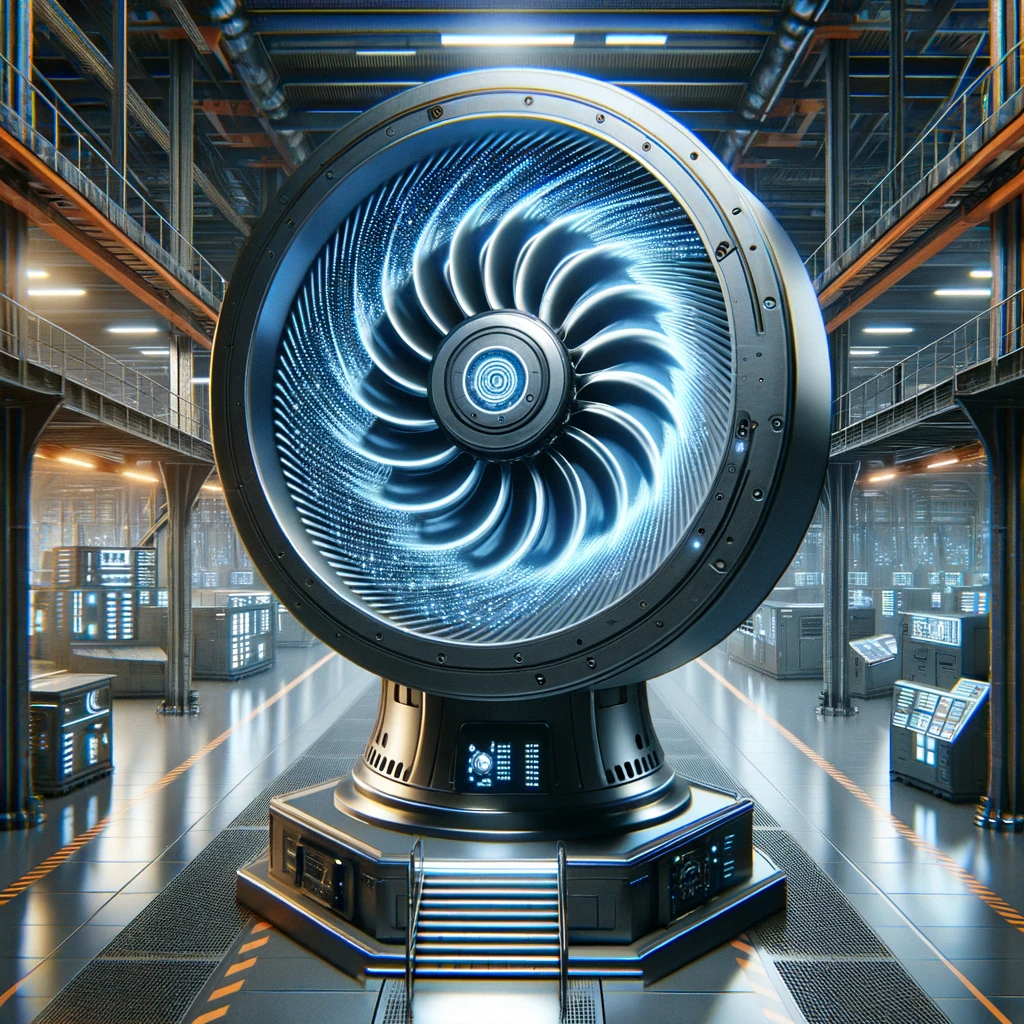
Back to Industrial Fans
In my personal opinion, I believe that the future design & simulation of industrial fans, as well as other turbomachinery and other not-so-bad-parametrizable machines, lies in the surrogate approach.
By its nature, a typical fan can be described with a set of parameters which allows straightforward classical simulations and building clear sets of training data. By utilizing machine learning, I believe that by 2030, we will be able to generate simulation-quality predictions in a matter of seconds, a significant improvement from the current time-consuming process that can take hours or even days.
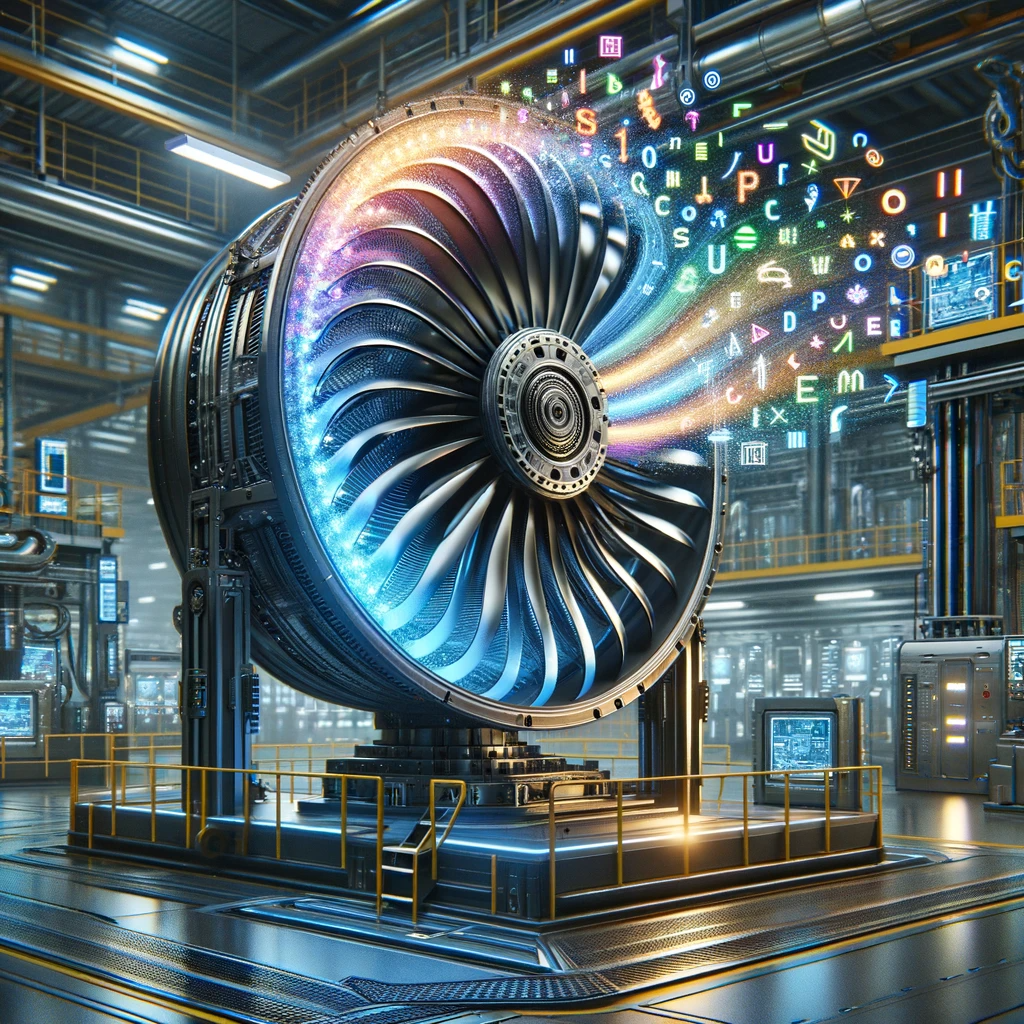
Things are already in motion
Company CFDSUPPORT is already developing & testing new software that helps engineers create their own knowledge databases and AI-based surrogate models. It’s expected to be released later this year. Ultimately, the goal is to create a platform where users can create their own custom databases (knowledgebases) and leverage their valuable know-how under their roof using the power of AI algorithms to improve their designs.
In a nutshell, I think AI models will totally take over the future of industrial CAE for typical simulations. Imagine getting simulation-quality predictions in just seconds! It’s gonna revolutionize the design process and save so much time and effort. With these advancements, we can expect better efficiency, performance, and innovation in computational-aided designs.
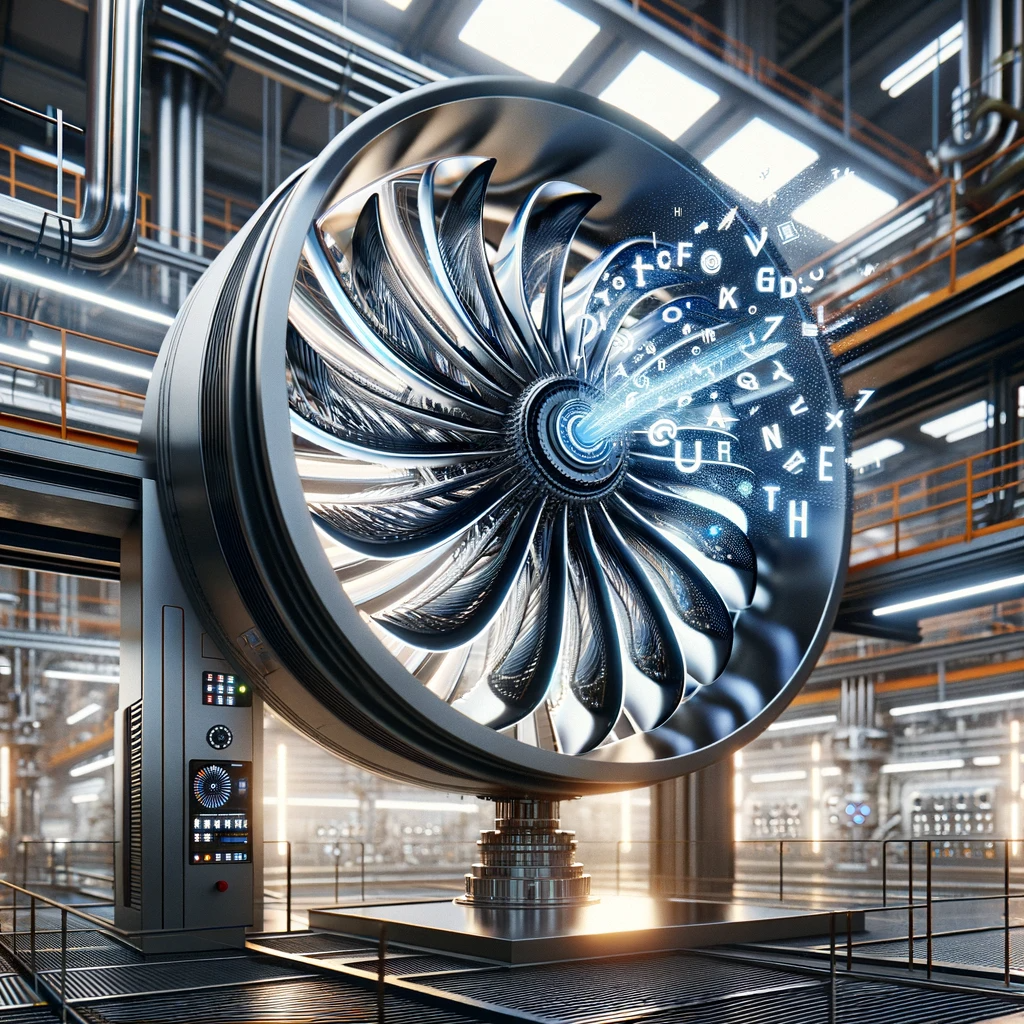
Disclaimer
AI will never completely replace the classical simulations. That is not possible by definition. There are many cases where AI just won’t work. It would be difficult to use AI for workflows with lack of training data. For example all the disposable projects or experimental and specific research projects. In fact, the demand for classical high-fidelity simulations could grow even further due to the increasing need for high-quality training data.
All in all
I’m believe that in the future, engineers will do fewer simulations but more analyze the data. The era of AI-aided CAE is on its way. Tthe question isn’t “ if”, but “how soon” it will happen.
Lubos Pirkl
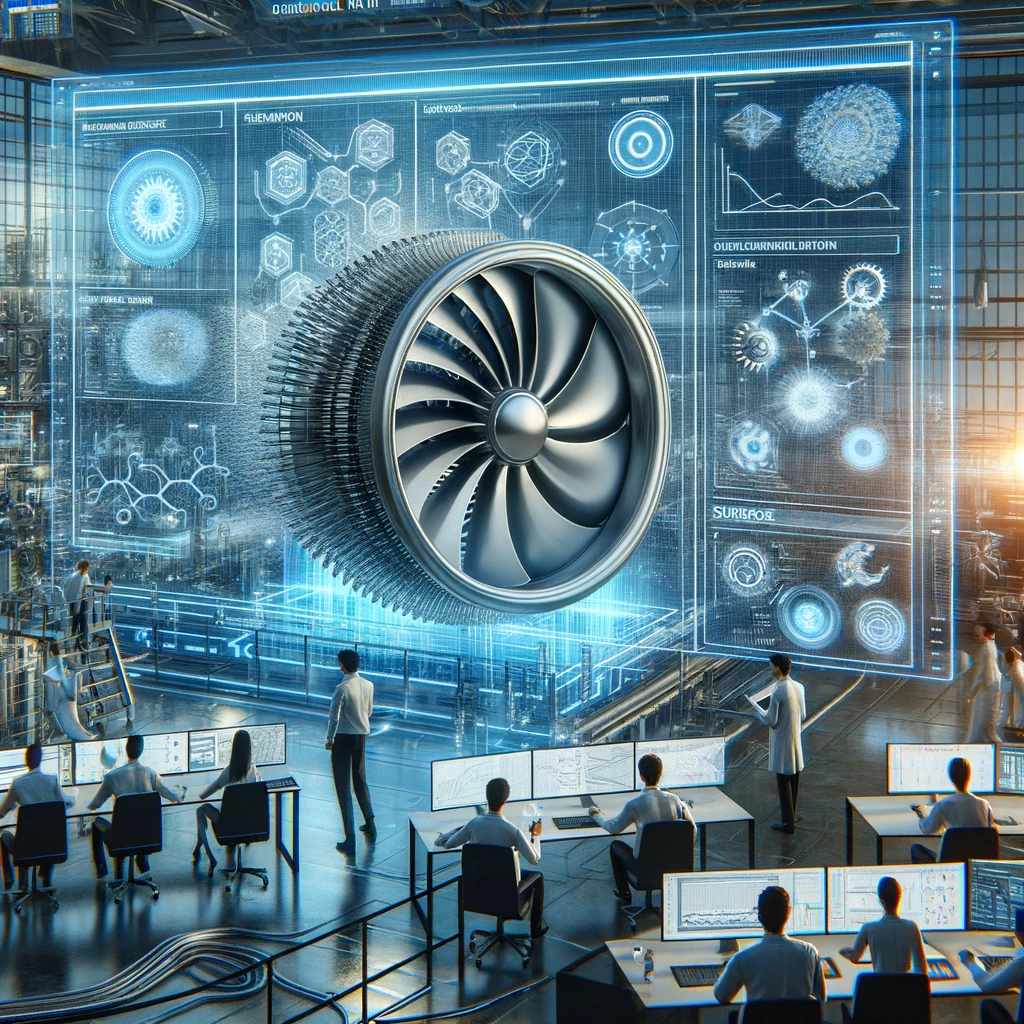
***The images (except the first one) featured in this article are computer-generated using DALL·E, a technology integrated into GPT-4, and should be noted as being purely fictional with no inherent significance.